Big Data is Not New
Today there is much talk about “big data”, with the assumption that this is a new and challenging area of analytics. Without a doubt the volume of data being analysed is greater now than ever before, but it is worth asking just how new the challenges really are.
In the 1880’s, Herman Hollerith was working at the US Census Bureau, heavily involved with the processing of census records that was carried out every ten years. He met the challenge of tabulating millions of census records by inventing an electromechanical system using punched cards. The cards were essentially a storage system for a big data set. The company Hollerith set up became today’s IBM.
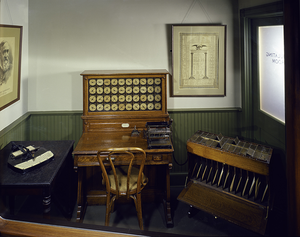
In the 1920’s, Ronald Fisher developed many of the statistical methods used today, when his computing power was limited to the Millionaire mechanical calculator. The Millionaire was very accurate – up to 20 decimal places – and fast for its time but had very limited storage, effectively two numbers. Fisher’s statistical methods made use of the Millionaire for problems such as the analysis of variance, especially for designed experiments. In those days a full analysis of an agricultural experiment with several hundred data points really was big data.
Fast forwarding to the 1960’s, statisticians were amongst the earliest to see the value of electronic computers. National statistical agencies such as the Australian Bureau of Statistics had amongst the largest mainframes available. New statistical algorithms were developed to make use of the new computers. For example, the origins of SAS lay in a new approach to fitting regression models. The 1970’s saw the rise of algorithm driven statistical methods such as generalised linear models.
Statistics has a critical role in modern big data problems, providing the theory to understand what new algorithms actually do, guiding the management of the data so that relevant information is retained and extracting insights and information. The final steps of any data analysis involves the classic statistical question “is it real or is it just chance?” It can be said that statistics puts the science into data science.
Data Analysis Australia has been at the forefront of practical big data since we began. In 1990, when “big” meant tens of megabytes, we worked with hundreds, utilising a Unix workstation and a network of PCs. In 2000, we worked with databases of over 100 gigabytes, and were developing parallel algorithms for record linkage tasks others thought were impossible. In 2010, we were delivering online monitoring tools using masses of weather data. Today our Spark cluster enables sophisticated statistical analysis of large datasets.
Data Analysis Australia is doing modern big data. But we are also continuing the tradition of statisticians who for over a century have combined the latest technology with theory to solve real problems.
For more information on how Data Analysis Australia can help you understand your data challenges, please contact <LINK daa@daa.com.au>daa@daa.com.au</link>.