Is it Real and is it Important?

It is human nature to see patterns in the world around us. Patterns in the shapes of clouds, rock formations, even in tea leaves. Today, in an era of ‘big data’, analysts are now more than ever trying to see patterns in large data sets.
The patterns we see aren’t always real, but the tendency to perceive them is a natural aid to survival. Consider a grazing antelope that hears a rustle in the grass – perhaps it’s the pattern of a lion’s stalk, or maybe just the randomness of the wind. For the antelope the choice is simple – if in doubt, run. For the same evolutionary reasons humans often see patterns, including in data, when none exist.
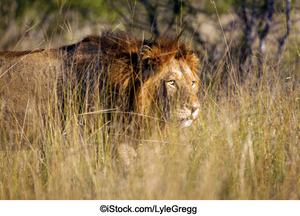
Statistics addresses this human failing, objectively assessing the available data, turning it into optimal action for all situations, not just those of the African savannah. Still, there are always error rates - false positives where patterns are accepted but are just coincidence, and vice versa for false negatives.
Of course, statistics isn’t simply the application of a set of black-and-white rules - blending statistical expertise with logic and reasoning is essential. The warning “correlation is not causation” is echoed in on-line examples showing how statistical techniques applied badly can identify relationships for things as unrelated as crude oil imports and the per-capita consumption of chicken. Similar logic and reasoning skills are required to determine if a ‘real’ pattern or relationship is actually important. Clearly the presence of a stalking lion is important to an antelope, but in a commercial environment, if a discovery won’t save time or money, then one might question the value of identifying the pattern in the first instance.
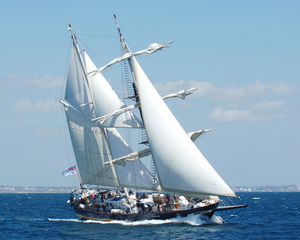
In recent years, increased storage capacity has led to a surge in data availability. With this comes increased potential for identifying patterns, whether they exist or not, and caution need be exercised. The information technology community manages and manipulates this large data. However, at Data Analysis Australia, our focus is not just on data management, but mining for meaningful, important patterns and information to make strategic decisions on data sets of all sizes.
Whilst the implementation needs to be adapted for large data sets, methods for extracting information are hardly new. Techniques such as regression, principal component analysis and cluster analysis are commonly known and are just a few of the devices in a statistician’s toolkit. As consultant statisticians, we also consider each problem in the context of our client’s business, ensuring a well-considered, sensible approach.
Data Analysis Australia’s work in this area is numerous and varied. One such project was the analysis of thousands of questionnaires from voyagers on-board the Sail Training Ship Young Endeavour. Exploratory analysis coupled with statistical data mining identified patterns relating voyage outcomes to factors such as demographics, experiences and weather conditions. For example, while durations varied, the most positive feedback came from participants in 12-day voyages. It was also discovered that lower satisfaction ratings for meals were almost always from participants in voyages that experienced strong winds! Insights such as this helped the client to ensure a more productive and enjoyable experience for all.
For further information on finding meaning in your data, please Contact Us.
March 2015