Identifying Cycles in a Chemical Plant
Overview
The Problem:
- To detect subtle long-term problems in a chemical plant using data obtained from a network of sensors which would have ordinarily been dismissed as unimportant.
The Data Analysis Australia Approach:
- To compare data from two similar plants, one with and one without signs of a fault, using principal component analysis to reduce the data to fewer variables and applying a time series analysis approach.
The Result:
- Temperature sensor readings were more distinct in one plant and cyclic behaviour was observed with a particular vaporising unit which directed attention to a section of the plant that, unbeknownst by Data Analysis Australia, the client was already suspicious of.
Background
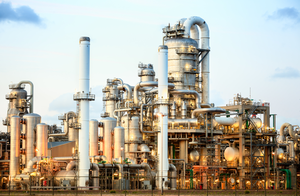
Modern chemical plants are immensely complicated with kilometres of piping connecting pumps, incinerators, reactors, slag collectors, and numerous other types of equipment. These in turn are monitored by hundreds of networked sensors which track conditions inside the plant and adjust the controls accordingly. All this provides continual surveillance of the plant’s operations and facilitates a rapid response when a component fails or a process breaks down.
At one time the data from such a network of sensors would have been discarded after its immediate use, but now, with storage so cheap, it can be kept. Can this data, measured in gigabytes or terabytes, also be used to detect more subtle, long-term problems?
The Problem
Data Analysis Australia was contacted by the owner of a production facility, where for more than fifteen years, one of the chemical plants had been experiencing drop-offs in production. These drop-offs required replacement of an expensive catalyst; the reduced production and the disruption to replace the catalyst were all real costs to the business. The client had contracted engineering firms to examine the plant’s components and operations to identify the problem, but to no avail. They therefore approached Data Analysis Australia to examine the problem from an entirely new angle - the data.
The Data Analysis Australia Approach
As with any exploratory project, it was impossible at the outset to predict what method of analysis would prove the most useful. Options examined by Data Analysis Australia for inclusion in the analysis plan all had to address three considerations:
- Due to the regularity of the time intervals at which readings were taken, the dataset naturally lent itself to time series analysis, which provided a frame of reference for the development of analytical ideas. However the length of the time series – hundreds of thousands of points – meant that many common time series methods could not be used.
- The hundreds of sensors meant many thousands of possible interactions had to be considered.
- The client was able to provide data from an almost identical co-located plant that was showing no signs of the faults causing production drop-offs that plagued the other.
After some investigation, Data Analysis Australia decided that this problem required looking at the data in terms of spectra, considering natural cycles in the data across a range of frequencies. Furthermore, the exploratory nature of the challenge suggested that Principal Component Analysis (PCA) should be applied to these spectra. PCA is a well known analytical tool that is used for dimensionality reduction – PCA takes data sets with many variables and returns combinations that account for the majority of the information. This allows us to “boil down” the many sensors in the data set to far fewer measures. PCA is normally used on non-temporal data, assuming all observations are independent of one another. Applying it to time series data, while not a completely new idea, is nonetheless not a common practice.
Using this analytic technique we were able to not only see which combinations of variables account for the most variation in the data set, but also how these combinations change as we looked at different frequencies, giving information on time lags in the plant. Subtle differences in the way the plants functioned became evident through the different compositions of the principal components for each plant.
The Result
Careful examination by Data Analysis Australia showed that, in plant 1, much of the first principal component was made up of the readings from temperature sensors, and this was the same across all frequency bands. Some distinct cyclic behaviour was also seen associated with a particular vaporising unit. In plant 2, on the other hand, these effects were far less pronounced, and the temperature sensor readings were less distinct from all other inputs. While this was not a smoking gun, it showed us a way in which these plants, while duplicated in design and co-located, were not behaving identically – and thus a potential connection to the underlying syndrome that Data Analysis Australia was looking for.
Outcomes
Data Analysis Australia’s analysis was presented to the senior engineers of the plant in question and general manager of the company. At this meeting, it became apparent that our independent analysis was directing attention to a section of the plant which, unbeknownst to us, the client was already suspicious of. Our report, said the general manager, was “the best dollars [they] had spent on this problem.”
June 2014