Profiles of Power
Overview
The Problem:
- Horizon Power needed to understand typical electricity consumption patterns of residential customers to assess the effect of potential changes in customer behaviour.
The Data Analysis Australia Approach:
- To simplify a large data set enabling the identification and understanding of different groups of customers within the data set, based purely on their electricity consumption and using a modelling approach to estimate the year-long pattern for each customer group.
The Result:
- A set of typical consumption patterns for different types of residential customers that can be used for ongoing ‘what if’ investigations of many different types of customer behaviour change to assist Horizon Power in their short and long term strategic planning.
The Problem
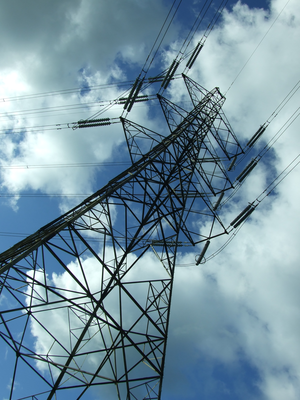
An electricity provider is expected by its customers to supply electricity at the flick of a switch, but how does the provider know when customers are going to flick that switch? Determining when the peak demand might occur and how high that peak might be requires an understanding of when different types of customers are likely to use electricity and how much they might use. Horizon Power therefore needed to develop a suite of “typical” daily consumption patterns or “load profiles” for their residential customers.
The Data Analysis Australia Approach
Based on our understanding of the key drivers of electricity consumption, we expected that the load profiles of residential customers would largely depend on the following questions:
- Does the household have air-conditioners installed? A household with an air-conditioner is likely to use significantly more electricity on hotter days.
- Does the household have solar panels installed? A household with solar panels is likely to purchase significantly less electricity during daylight hours, when they can supply their own.
- Does the household have people at home during the day on weekdays? A household with people at home during the day is likely to use more electricity in the middle of the day, especially if they have an air-conditioner.
However, electricity providers don’t usually know whether each household they must supply has an air-conditioner and they certainly don’t know whether there is likely to be someone at home during the day. Therefore Data Analysis Australia’s approach was to work with raw electricity consumption data to try to identify daily consumption patterns that might match our expectations.
We used half-hourly electricity consumption for thousands of individual customers; data that were collected over a time period spanning several years as part of Western Power's Perth Solar City program. As there are 8,760 hours in a year, the data set contained hundreds of millions of cells and millions of records. From this we wanted to obtain a relatively small number of load profiles.
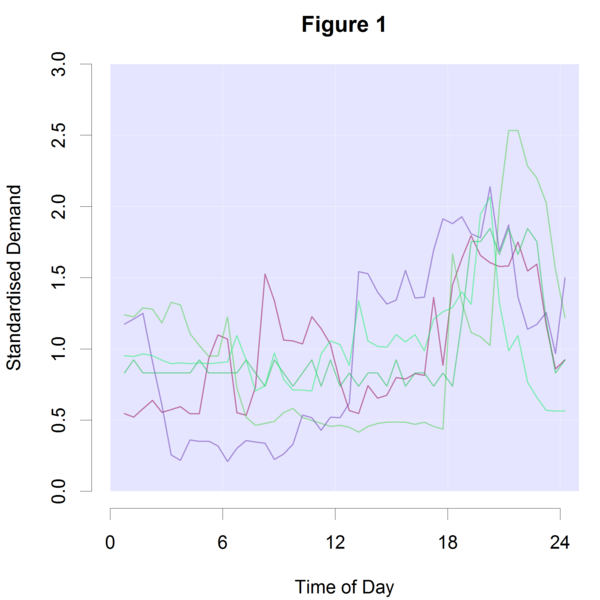
Recognising that electricity consumption is a very “noisy” process, with a lot of random variation from hour to hour (see Figure 1 - actual electricity usage for a single day for a sample of customers), we considered that averaging the data would achieve two things:
1. Reduce the number of records; and
2. Remove some of the random variation.
Both of these outcomes would make it easier to recognise and separate the underlying patterns.
Before averaging the data we needed to carefully consider the appropriate way to average. Electricity usage is likely to be different on weekdays and weekend days for many households, with people being out at work and school on weekdays. As a large proportion of a household’s electricity usage is often due to appliances such as refrigerators, heaters and air conditioners, patterns are also likely to vary between summer and winter. Therefore, rather than simply averaging over all days of the year, we determined that it was most appropriate to average the raw consumption for each customer by time of day separately for weekdays and weekends and for each season.
By this stage the size of the data set was quite manageable and the real analysis could begin. Using statistical techniques such as cluster analysis, and feeding in each customer’s averaged daily profile it was possible to group customers with similar usage patterns across the day. Further averaging was then undertaken across all customers within an identified group. This enabled us to review the groups’ profiles for meaning and to determine how many different profiles were really needed. While clustering techniques can automate the grouping process, our background knowledge and understanding enabled us to recognise the type of household that would be expected to exhibit a particular pattern and hence make decisions regarding the number of clusters to utilise.
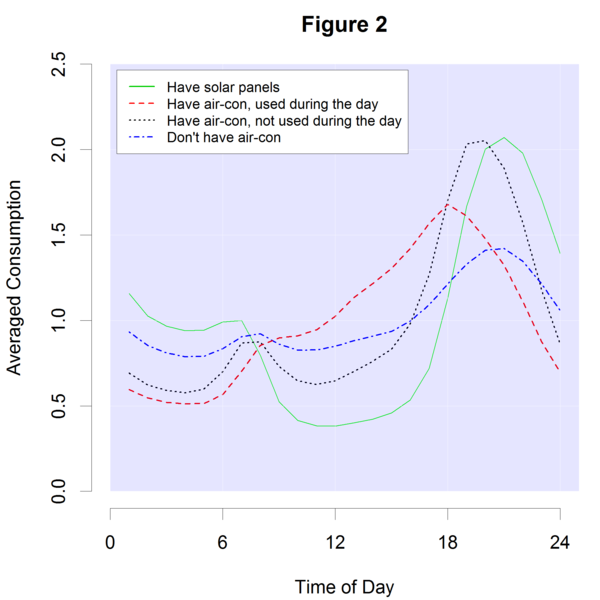
Four understandable profiles were obtained from the clustering approach (see Figure 2 - averaged electricity usage for customer groups):
- Households with solar panels installed and hence very low consumption during the day;
- Households with air-conditioning used all day long;
- Households with air-conditioning used only in the mornings and evenings; and
- Households with no air-conditioning.
While a relatively simple averaging process allowed us to tease out the underlying patterns in the data set, a more sophisticated analysis was required to obtain a year long profile for each group. Such profiles would then be used to facilitate additional analysis to answer the question “how much power is likely to be used at any given time of day on any given day of the year?”. This sophisticated approach required returning to the original data set, before any averaging was done, but incorporating the group to which each customer had been assigned.
Statistical models were fitted using the raw data and including external information, such as whether a given date was a weekend day, a Public Holiday and/or during school holidays, plus actual weather for each day. By understanding how much power was consumed under particular weather conditions the models were then used to predict how much power would be consumed under “typical” weather conditions, and hence a “typical” consumption profile could be determined for each profile group.
The Result
A suite of residential customer year-long power consumption profiles were obtained, each of which could be understood in terms of particular customer behaviours. These profiles provided a basis for further analysis to investigate potential changes in customer behaviour and the likely effect of such changes on the power grid. For example, if 20% more customers were to install air-conditioning, what would be the likely effect on the amount of power that would have to be generated to cope with this increased demand? Data Analysis Australia has continued to work with Horizon Power to analyse and understand several different scenarios, including the effect of having large numbers of customers installing solar panels.
March 2015