Mining Analytics
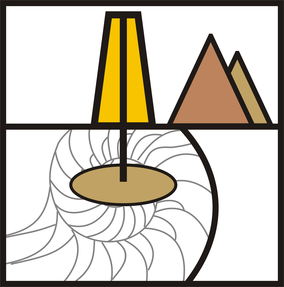
Data Analysis Australia has a long history of working with clients from across the mining, oil and gas sectors to help them gain new insights from their existing data resources and to design and inform effective data collection strategies. As consultant statisticians with experience across many industries and sectors we utilise not only statistical techniques that have proven applications in mining but also adapt techniques traditionally used in other sectors to provide innovative solutions to mining challenges.
Techniques used by Data Analysis Australia’s statisticians for mining applications include:
Regression modelling, including generalised linear models and semi-parametric regression;
Predictive modelling and forecasting;
Model selection algorithms and cross-validation;
Geostatistics and spatial analysis;
Simulation and optimisation;
Cluster analysis to understand groupings amongst ore samples;
Classification and Regression Trees to model key variables by partitioning a data set; and
Experimental design for mineral grade, recovery and hardness testwork programs.
Related Case Studies
Electrowinning
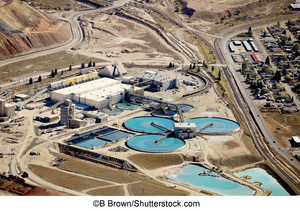
The Problem: A mining company needed to understand and resolve a change in the current efficiency of the electrowinning circuit at one of their mines.
The Data Analysis Australia Approach: Through the use of a range of statistical tools, including breakpoint analysis and autoregressive linear models, the available data for the circuit were analysed. After the changes in the current efficiency over time were understood, Data Analysis Australia investigated a range of potential causes.
The Result: Breakpoint analysis demonstrated that the most recent change in efficiency was beyond previous trends and variability. Investigation of the circuit’s efficiency leading up to this “break” identified an irregular event that preceded the efficiency changes. The client was then able to identify a particular sequence of events and take action to improve efficiency and prevent instability in the electrowinning circuit.
Identifying Cycles in a Chemical Plant
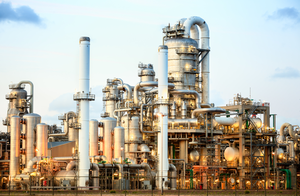
The Problem: To detect subtle long-term problems in a chemical plant using data obtained from a network of sensors which would have ordinarily been dismissed as unimportant.
The Data Analysis Australia Approach: To compare data from two similar plants, one with and one without signs of a fault, using principal component analysis to reduce the data to fewer variables and applying a time series analysis approach.
The Result: Temperature sensor readings were more distinct in one plant and cyclic behaviour was observed with a particular vaporising unit which directed attention to a section of the plant that, unbeknownst by Data Analysis Australia, the client was already suspicious of.
Statistical Data Science
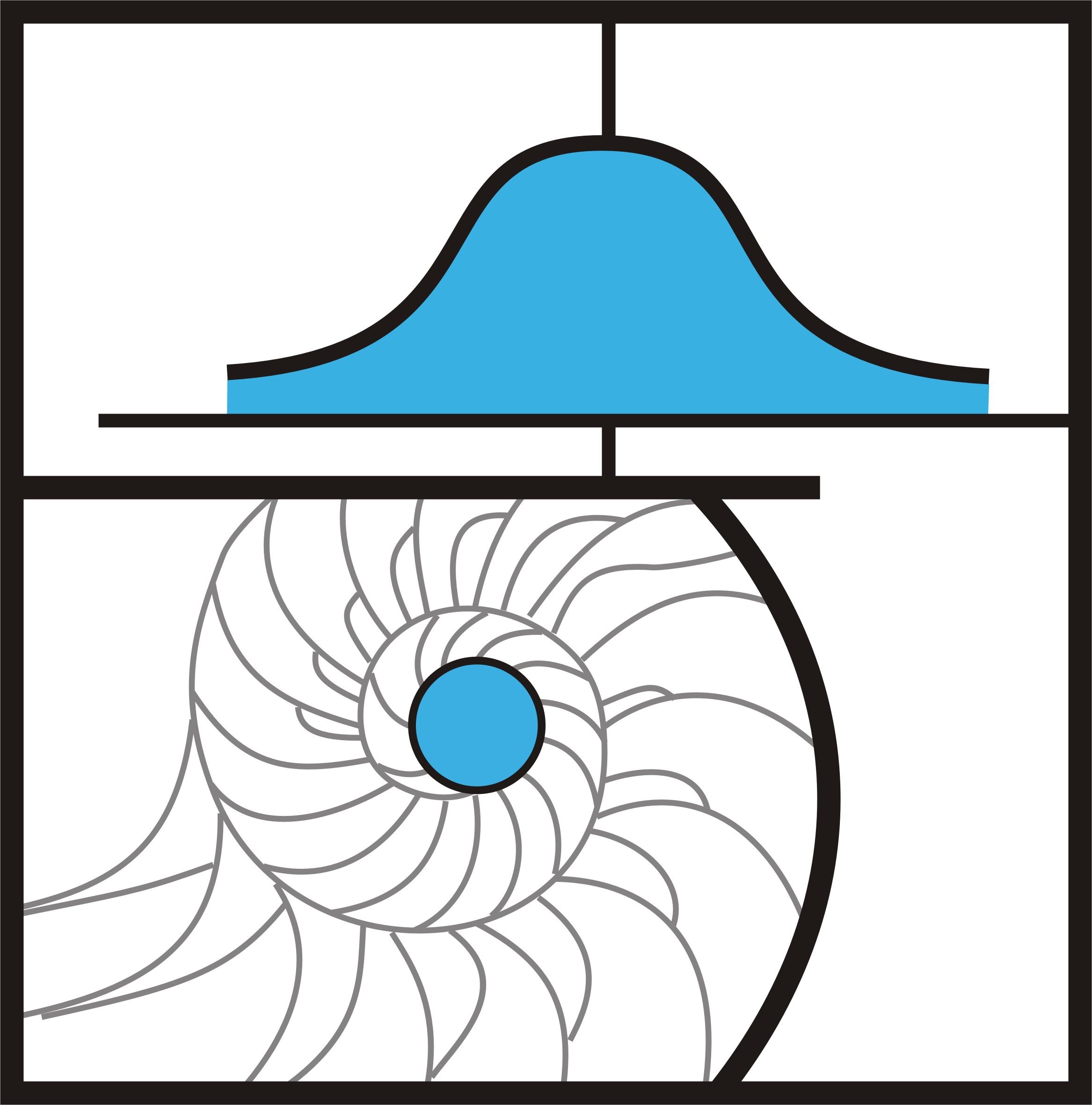
Analyse, visualise, and model data using the latest statistical and data science techniques
Surveys
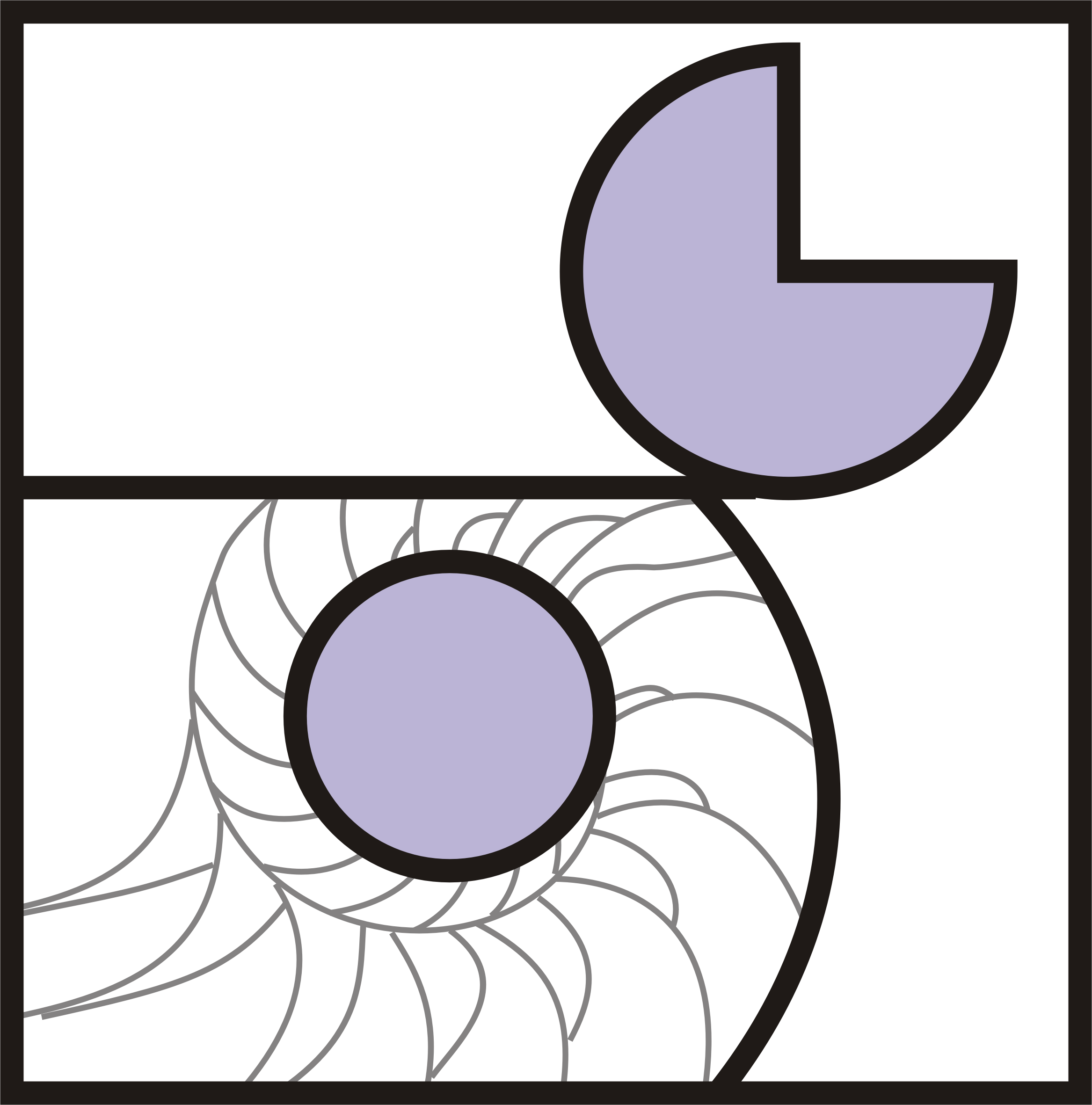
Develop, carry out and analyse surveys to understand perception and find business insights
Forecasting and Prediction
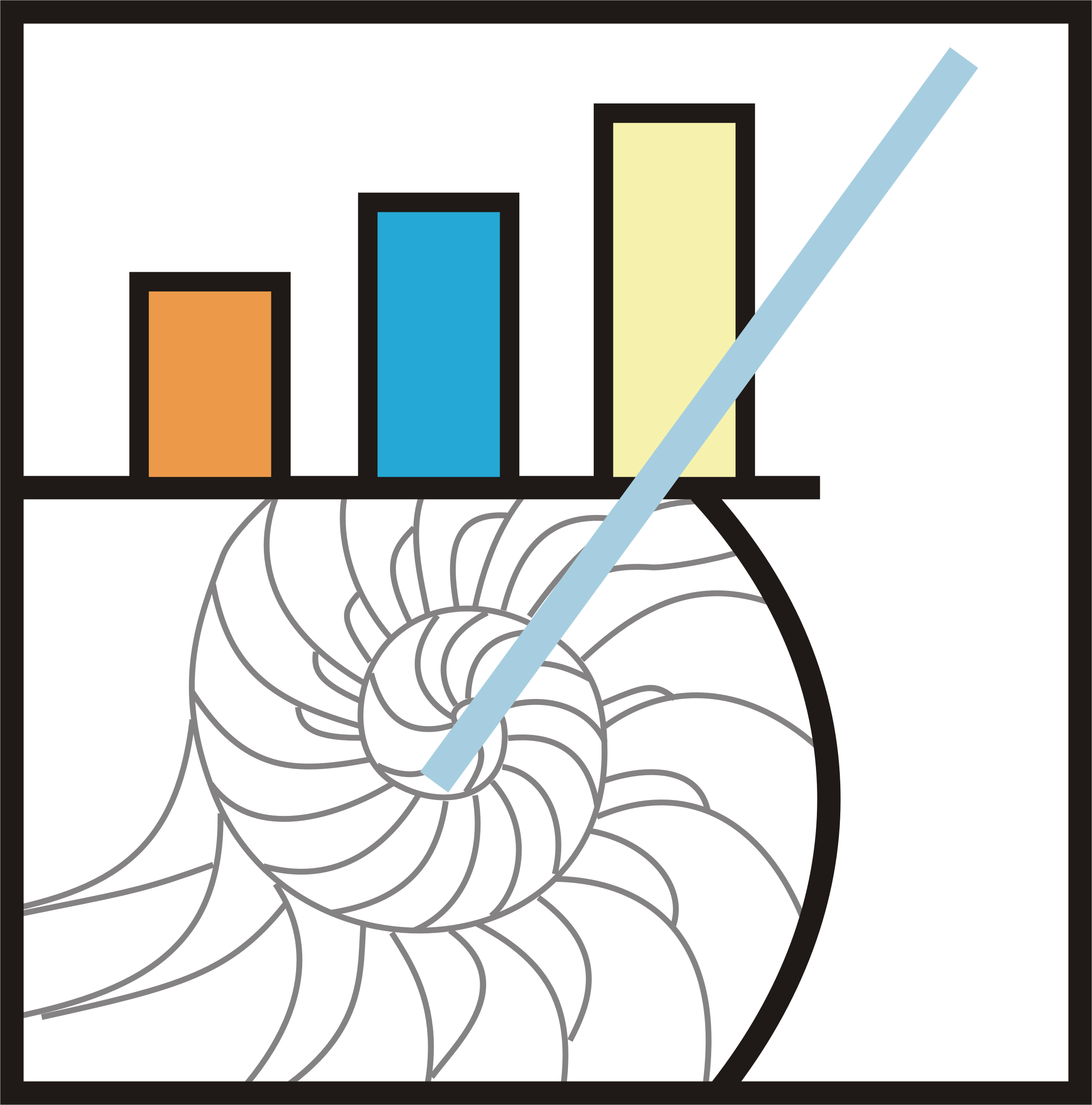
Discover trends and predict the future with data
Spatial Analysis and Mapping
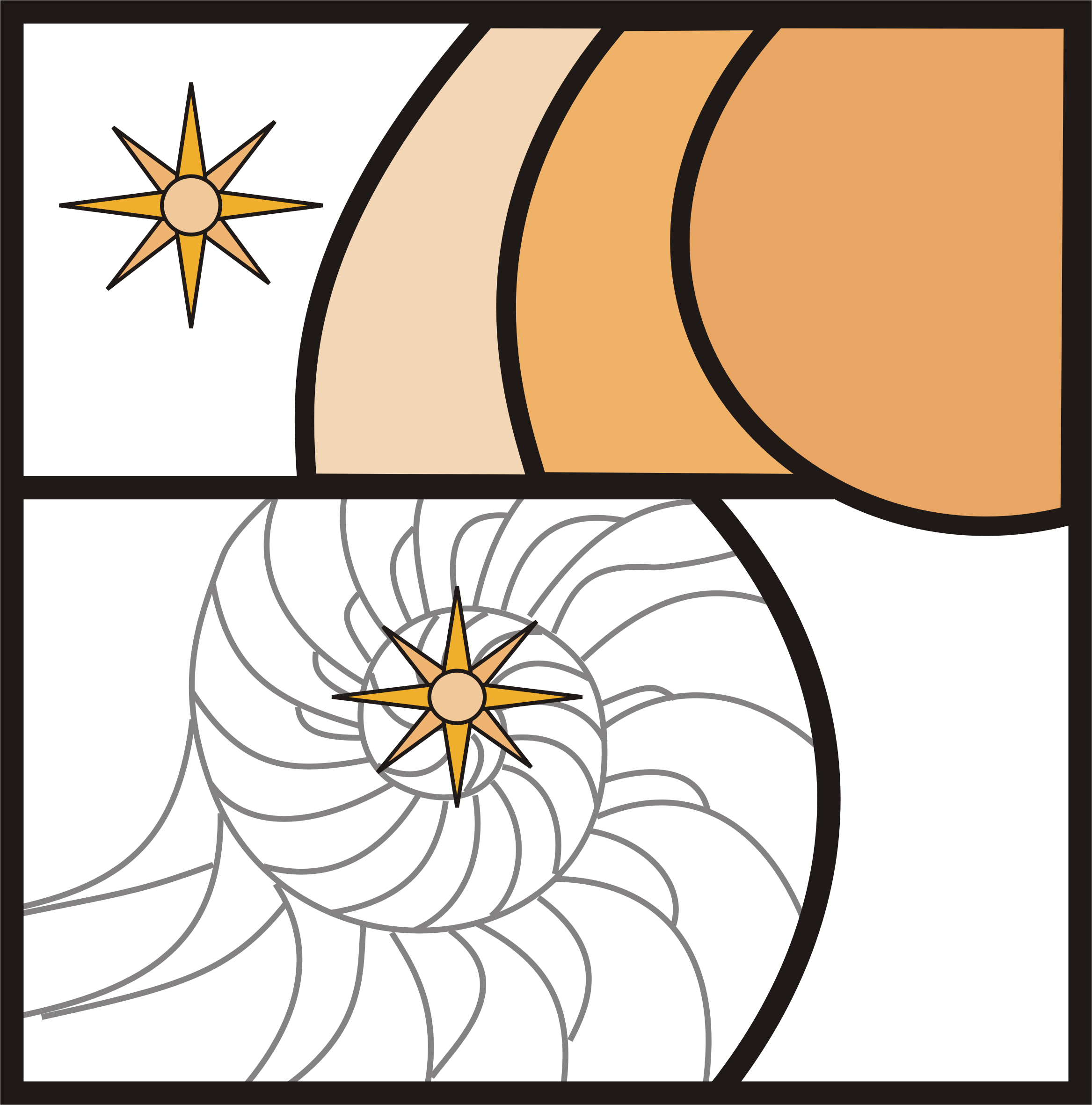
Learn how data is spatially correlated to inform strategy
Business and Risk Analysis
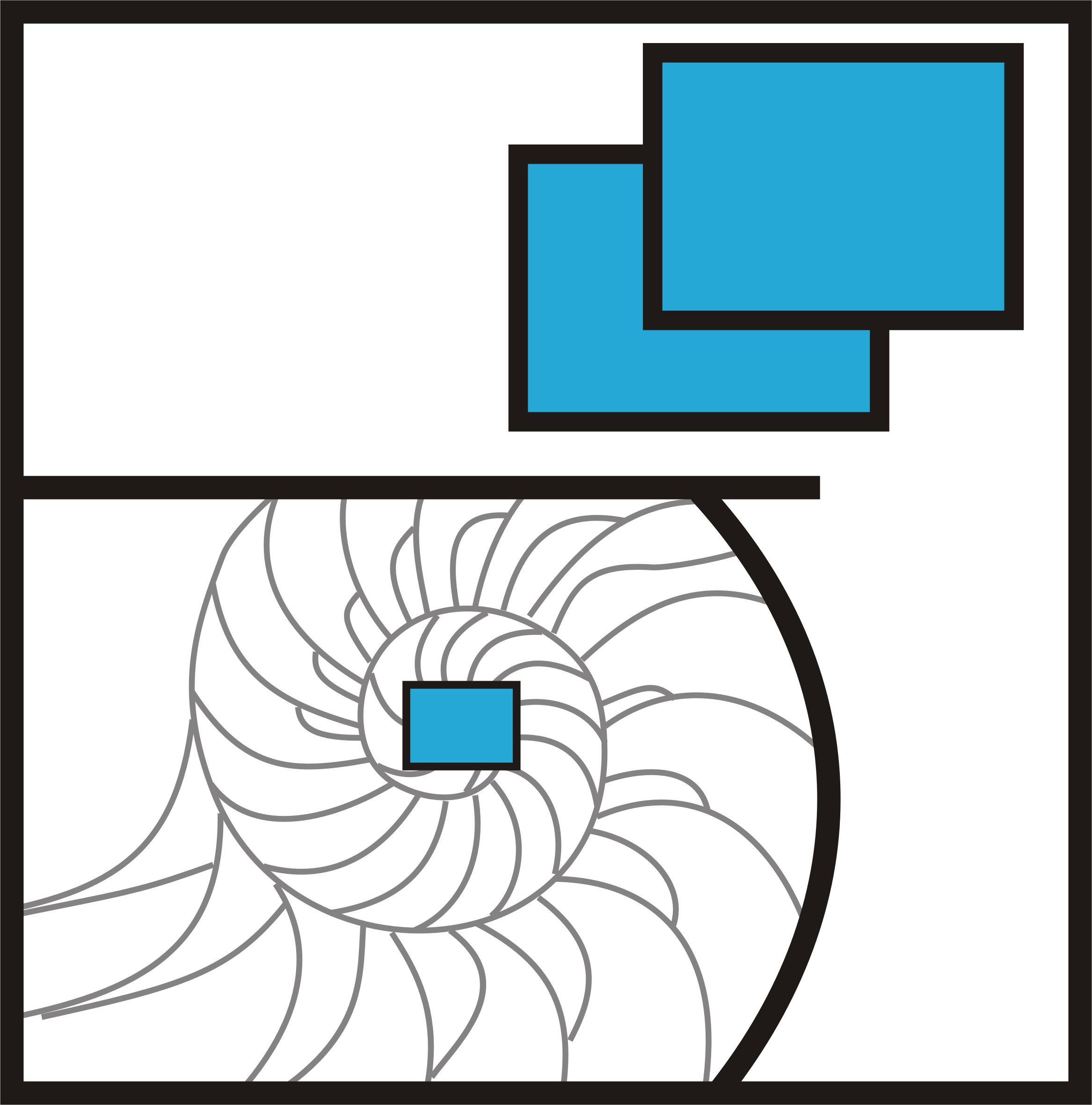
Understand how risk and uncertainty can be minimised in business decisions
Big Data and Machine Learning
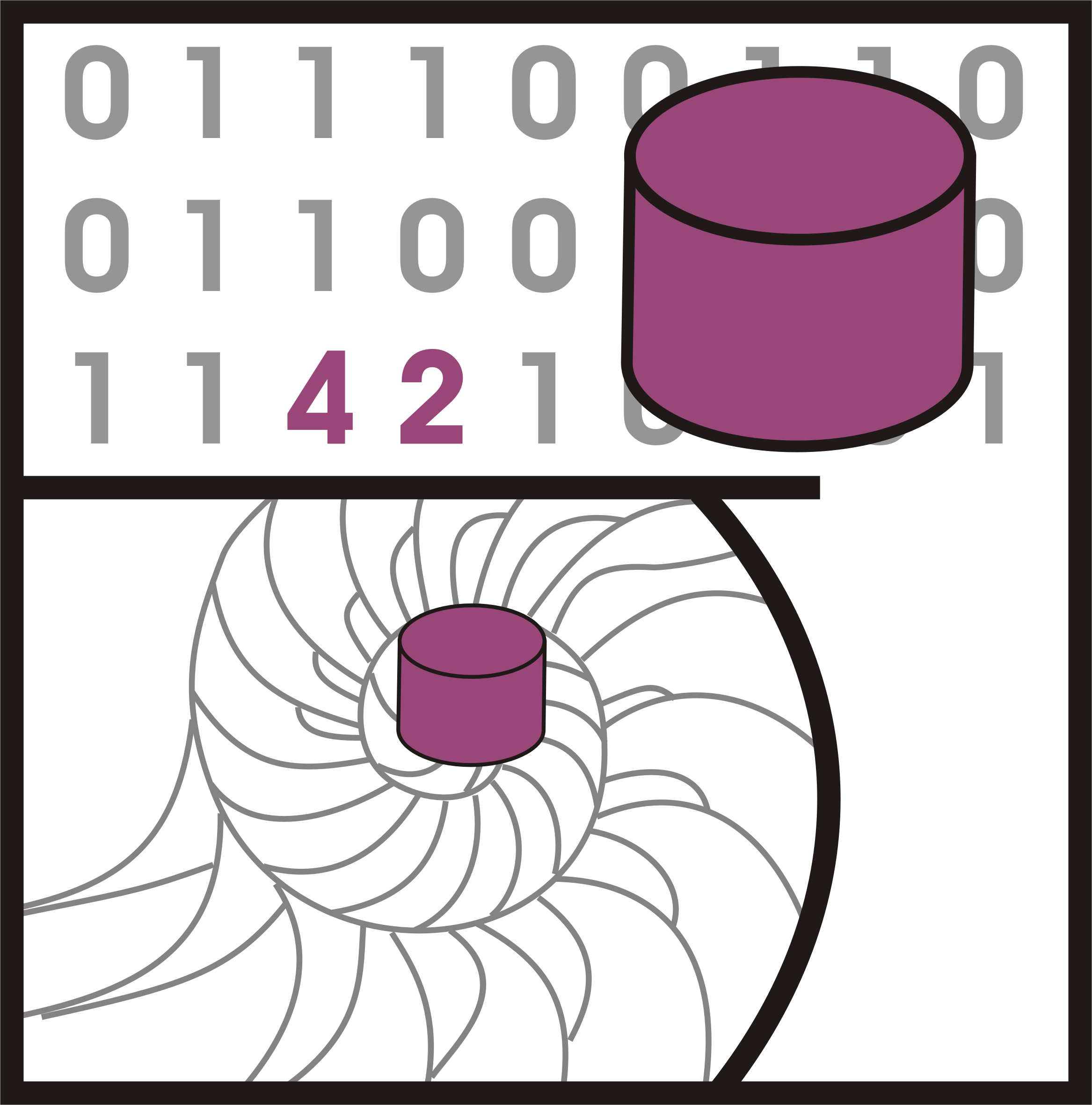
Uncover trends and relationships to gain valuable insights from big data
Simulation and Optimisation
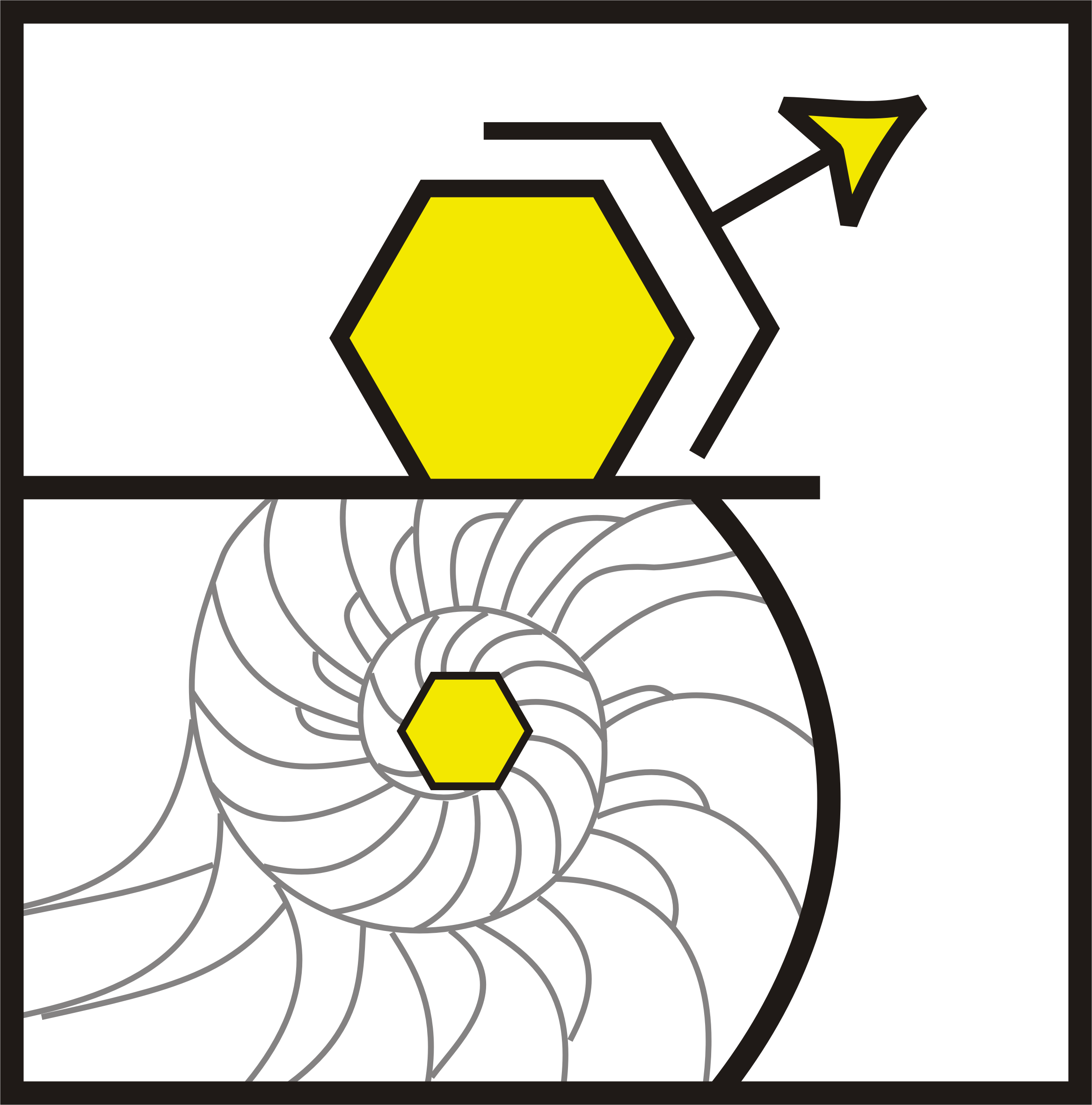
Determine the optimal way to operate in the future
Mining Analytics
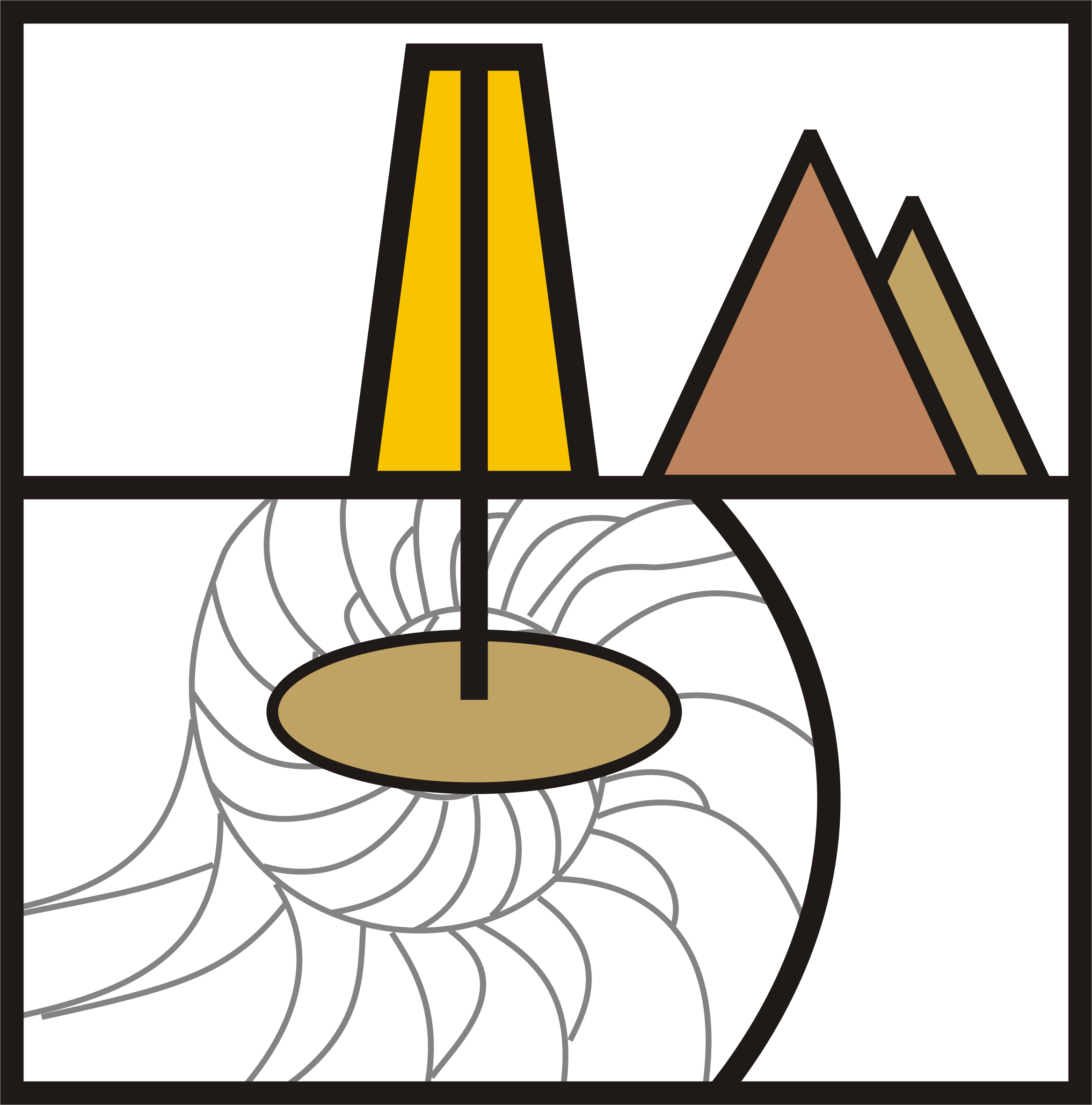
Improve processes and uncover new insights
Interactive Dashboards
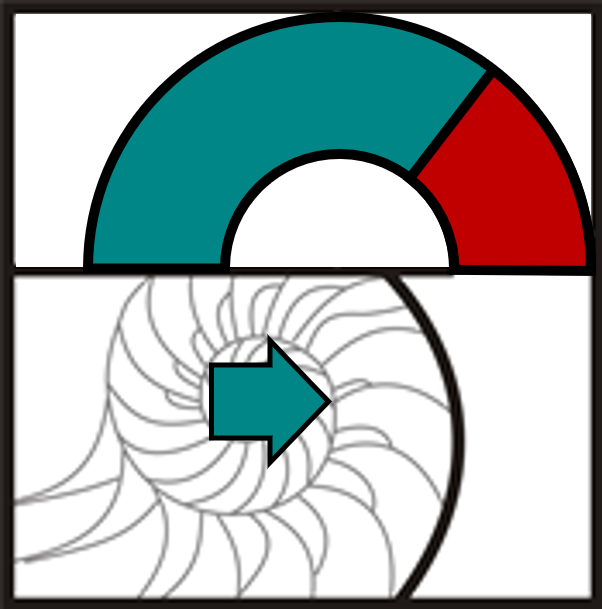
Make informed decisions from real-time data with intuitive visuals and information